Imagine you’re a data scientist, ready to dive into the world of AI and deep learning. You need a GPU that can handle massive datasets, complex neural networks, and high-performance computing. But with NVIDIA’s lineup of powerful GPUs, which one should you choose? The A100, the latest in GPU technology, or the tried-and-true V100? This decision can make or break your project’s success.
The world of AI, data analytics, and high-performance computing (HPC) is ever-evolving, and choosing the right tools is critical. NVIDIA has long been at the forefront of GPU innovation, pushing the boundaries of what’s possible in computing. Their GPUs are no longer just for gaming; they are the backbone of modern AI and HPC. In this blog, we’ll explore the differences between the NVIDIA A100 and V100 GPUs, helping you decide which one is the best fit for your needs.
The Evolution of GPU Technology
GPUs were initially designed to handle graphics rendering, making them essential for gaming and visual media. However, as AI and HPC became more prevalent, GPUs were repurposed to handle tasks that CPUs couldn’t manage effectively. NVIDIA has been a pioneer in this transformation, creating GPUs that cater to the diverse needs of today’s computational demands.
NVIDIA V100: The Veteran in the Field
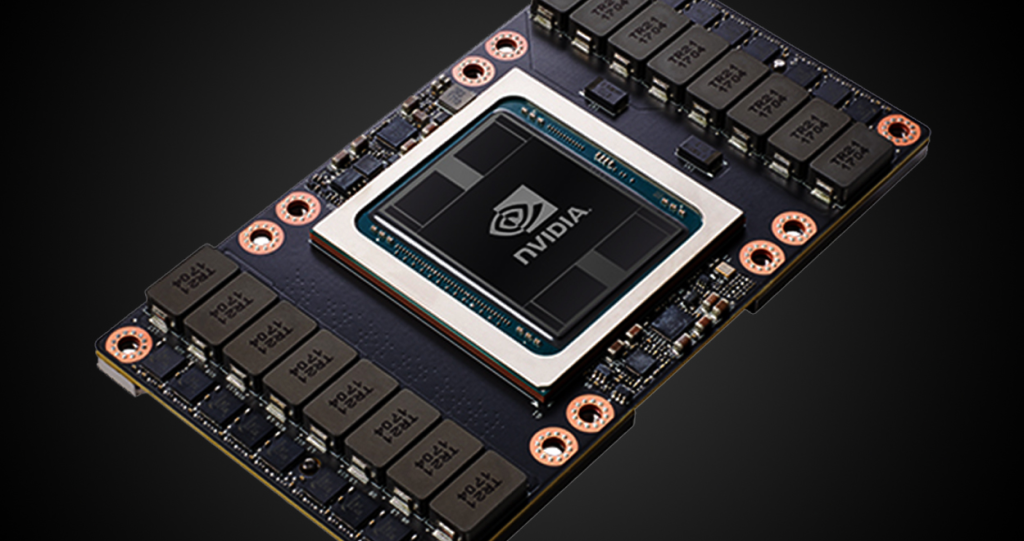
Introduced in 2017, the NVIDIA V100 was a game-changer in HPC and AI acceleration. Built on the Volta architecture, it brought innovations like Tensor Cores that significantly boosted AI and deep learning performance. With 5,120 CUDA cores and up to 32GB of HBM2 memory, the V100 quickly became a favorite for data centers and research institutions.
The V100’s Volta architecture also introduced independent thread scheduling, optimizing parallel efficiency by reducing penalties associated with divergent code execution. This was a significant leap from previous architectures like Pascal, where thread synchronization could lead to underutilization of GPU resources.
NVIDIA A100: The New Titan of AI
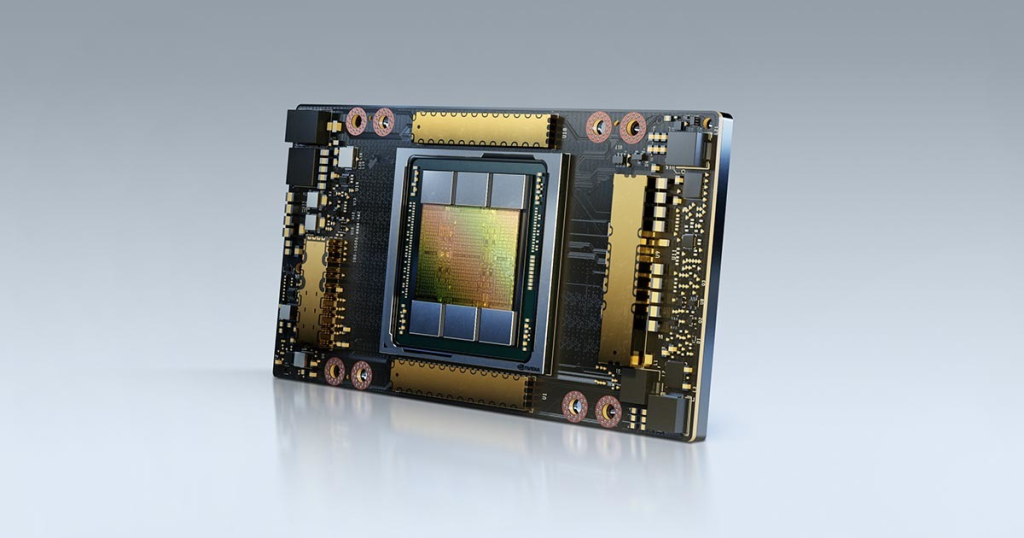
In May 2020, NVIDIA introduced the A100, built on the Ampere architecture, which represents a substantial upgrade over the V100. The A100 is designed for the next generation of AI and HPC workloads, offering up to 40GB of HBM2e memory and 6,912 CUDA cores. Its performance improvements, such as structural sparsity and Multi-Instance GPU (MIG), make it a powerhouse for data centers and large-scale AI projects.
Structural sparsity allows the A100 to skip over zeros in datasets, effectively doubling its processing speed for AI tasks. Meanwhile, MIG enables the A100 to be partitioned into smaller GPUs, each capable of handling different tasks independently. This flexibility is invaluable in environments where multiple users or tasks need to share GPU resources efficiently.
Head-to-Head: A100 vs. V100 Performance
When it comes to raw performance, the A100 outshines the V100 in almost every category. It delivers up to 156 teraflops (TFLOPS) of single-precision performance, compared to the V100’s 15.7 TFLOPS. For AI tasks, the A100 can reach an astonishing 312 TFLOPS using structural sparsity, while the V100 caps at 125 TFLOPS.
Here’s a breakdown of their specs:
Specification | NVIDIA A100 | NVIDIA V100 |
---|---|---|
Architecture | Ampere | Volta |
CUDA Cores | 6,912 | 5,120 |
Tensor Cores | 432 (3rd Gen) | 640 (2nd Gen) |
Memory | 40GB HBM2e | 16/32GB HBM2 |
Memory Bandwidth | 1.6 TB/s | 900 GB/s |
FP32 Performance | 156 TFLOPS | 15.7 TFLOPS |
TDP | 400W | 300W |
Target Market | AI, HPC, Data Analytics | AI, HPC, Scientific Research |
Application Suitability: Where Each GPU Excels
AI and Deep Learning
The A100 is the go-to for large-scale AI training and inference. Its enhanced Tensor Cores and structural sparsity support make it ideal for complex neural networks and data-intensive tasks.
Scientific Research and HPC
Both GPUs excel in scientific computing, but the A100’s higher memory bandwidth and CUDA cores make it better suited for the most demanding simulations and research projects.
Cloud Computing and Data Centers
The A100’s ability to handle multiple tasks simultaneously, thanks to MIG, makes it a superior choice for cloud environments where scalability is key. However, the V100 remains a solid option for organizations with less intensive workloads.
Cost and Value Analysis
The A100 is more expensive than the V100, reflecting its newer technology and higher performance. However, when considering the total cost of ownership, including energy efficiency and future-proofing, the A100 may offer better long-term value, especially for large-scale deployments.
Future-Proofing: Is the A100 Worth the Investment?
With AI and HPC workloads becoming more complex, future-proofing your infrastructure is essential. The A100, with its advanced architecture and features, is better equipped to handle the evolving demands of AI and data analytics. NVIDIA’s ongoing support and updates for the A100 will likely extend its lifespan, making it a wise investment for those looking to stay ahead of the curve.
Conclusion: Which GPU Should You Choose?
Choosing between the NVIDIA A100 and V100 depends on your specific needs and budget. If you’re working on cutting-edge AI projects or need the highest possible performance for HPC, the A100 is the clear winner. However, the V100 still offers excellent value for less demanding tasks and can be a cost-effective solution for many organizations.
In the rapidly changing world of AI and HPC, staying informed about the latest GPU technologies is crucial. Whether you choose the A100 or V100, both GPUs offer incredible capabilities that can help you achieve your computational goals.
Leave a Reply
You must be logged in to post a comment.