Choosing the right GPU for AI, machine learning, and data center workloads is crucial for optimizing performance and efficiency. The NVIDIA A30 and A40 GPUs are among the top choices for these purposes, offering unique features tailored to different use cases. This post will compare the two, helping you decide which GPU best suits your needs.
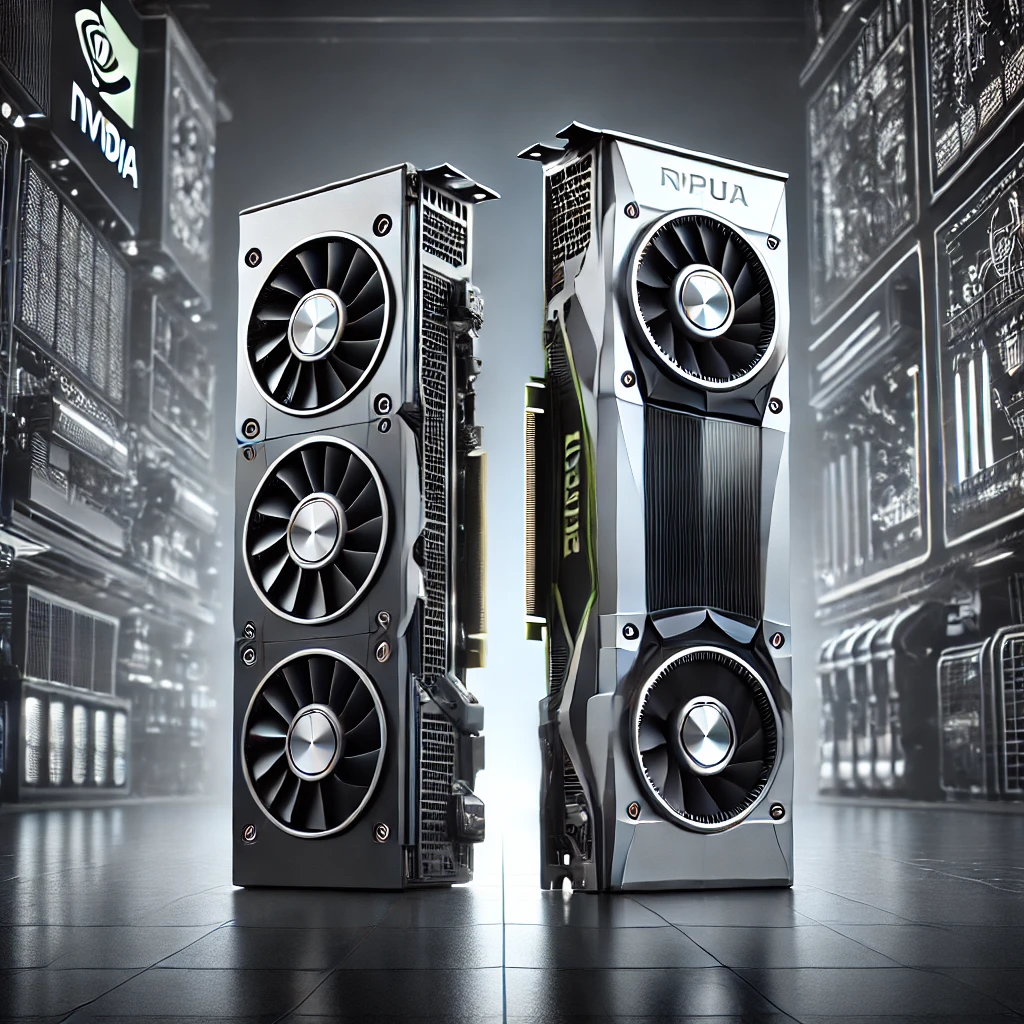
Advanced Performance Features of the A30 and A40
The NVIDIA A30 and A40 are both engineered to address the growing demand for specialized computing power in AI, HPC, and visualization, but they each shine in different areas of application. One of the most distinguishing features of the A40 is its high CUDA core count, making it an exceptional choice for professionals working with large-scale visualization tasks like 3D rendering, medical imaging, and video production. The A40’s large memory capacity (48GB of GDDR6) enables users to work with larger datasets, making it a powerful tool for complex simulations or visualization workloads that require significant computational power.
On the other hand, the A30’s optimized design for AI workloads is particularly noteworthy. It excels in mixed precision tasks, especially in FP16, which is crucial for AI training and inference applications. With 165 TFLOPS in FP16 performance, the A30 is optimized for high-throughput AI workloads like deep learning model training, natural language processing (NLP), and computer vision. Its lower power consumption, at 165W, allows businesses to scale their AI infrastructure efficiently while minimizing operational costs.
Another important consideration is memory bandwidth. The A30’s 933 GB/s bandwidth, which outpaces the A40’s 696 GB/s, allows for faster data throughput in AI and machine learning tasks where speed is critical. This gives the A30 an advantage in environments requiring fast, data-intensive processing.
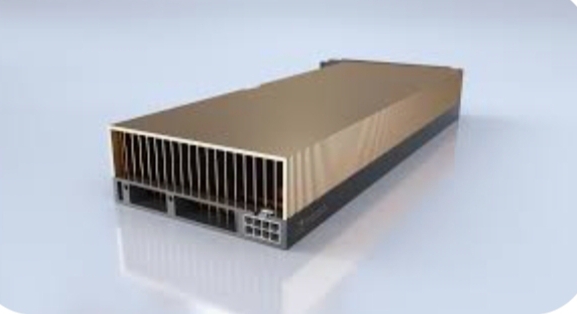
Overview of NVIDIA A30 and A40
Here’s an overview of the NVIDIA A30 and A40 GPUs, focusing on their specifications and target applications:
- NVIDIA A30
- Architecture: Ampere
- Target Use: AI inference, machine learning, and high-performance computing (HPC)
- Memory: 24 GB GDDR6
- TDP (Thermal Design Power): 165W
- Performance: Optimized for smaller-scale AI workloads, such as AI inference and edge computing.
- NVIDIA A40
- Architecture: Ampere
- Target Use: AI training, rendering, and high-performance computing
- Memory: 48 GB GDDR6
- TDP: 300W
- Performance: Designed for larger-scale AI model training and complex data center workloads that require more memory and computational power.
Key Specifications Comparison
Specification | NVIDIA A40 | NVIDIA A30 |
---|---|---|
CUDA Cores | 10,752 | 6,912 |
Tensor Cores | 336 | 276 |
FP32 Performance | 19.2 TFLOPS | 10.3 TFLOPS |
FP16 Performance | 78 TFLOPS | 165 TFLOPS |
Memory Size | 48GB GDDR6 | 24GB HBM2 |
Memory Bandwidth | 696 GB/s | 933 GB/s |
Power Consumption (TDP) | 300W | 165W |
Performance Insights
- Single-Precision (FP32) Performance: The A40 outperforms the A30 in FP32 tasks, making it better suited for visualization-heavy applications such as rendering and complex simulations.
- Mixed Precision (FP16): The A30 excels in FP16 operations, which are crucial for AI training and inference workloads, offering a significant advantage in deep learning scenarios.
Memory and Bandwidth
- A40: With 48GB of GDDR6 memory, it is tailored for tasks that require substantial memory capacity, such as large-scale simulations or visualization projects.
- A30: Offers 24GB of HBM2 memory, optimized for rapid data access, making it perfect for AI training, inference, and HPC workloads that need efficient memory bandwidth.
Energy Efficiency
Energy efficiency is a critical factor, especially in data center environments:
- A40: Rated at 300W, this GPU requires robust cooling solutions due to its higher power draw.
- A30: With a TDP of only 165W, it is designed for energy efficiency, making it suitable for data centers with limited cooling capabilities.
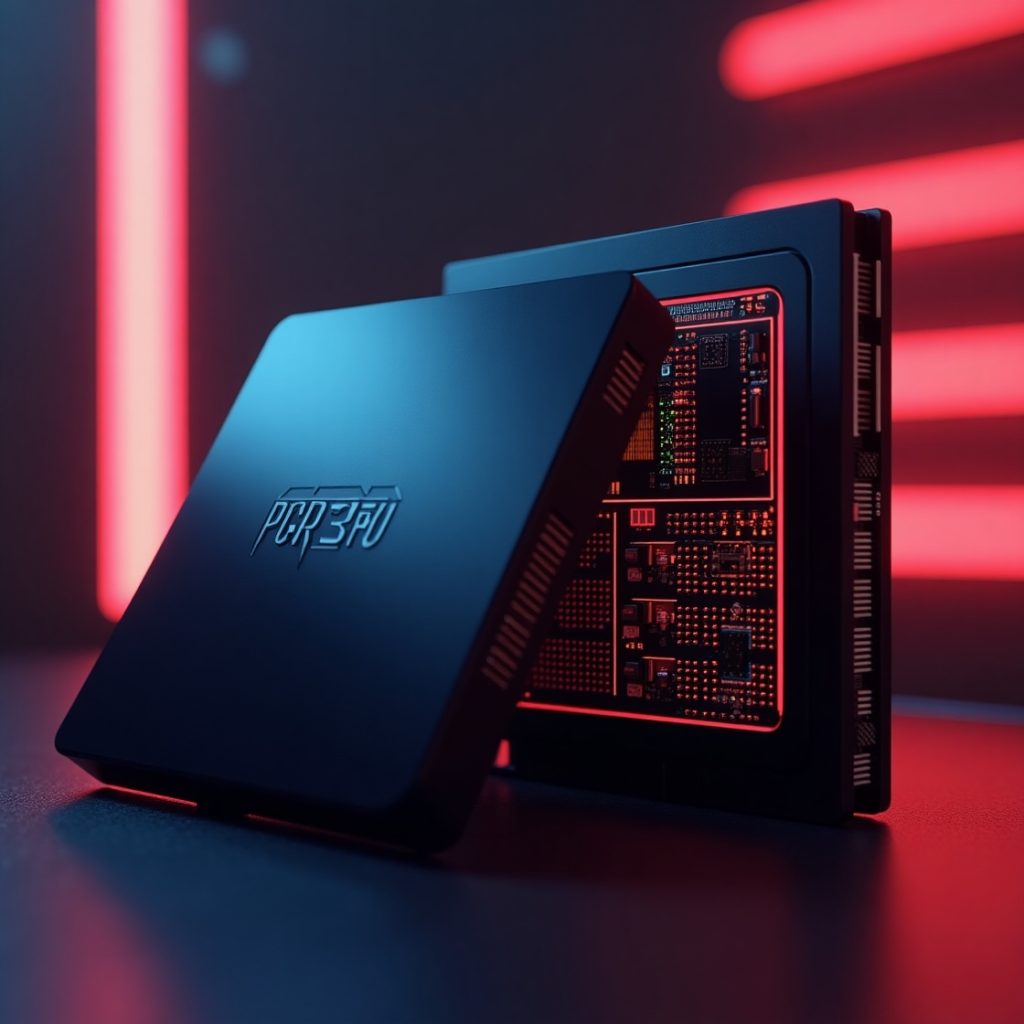
Which GPU Should You Choose?
Now that we’ve outlined the key differences, it’s time to guide you on which GPU to choose based on your specific needs:
- For AI Inference and Cost-Efficiency: If your focus is on AI inference, edge computing, or smaller-scale AI applications, the A30 offers the best balance between price and performance, making it a more affordable choice for enterprises with moderate workload demands.
- For AI Training and Heavy Workloads: For larger AI model training, complex data processing, or HPC applications, the A40 is the better choice due to its larger memory, superior performance, and ability to handle more demanding tasks.
Conclusion
In conclusion, both the NVIDIA A30 and A40 are excellent GPUs for AI and data center workloads, but they serve different purposes. If you need a GPU for cost-effective AI inference or edge computing, the A30 is a great option. On the other hand, if you require powerful computational resources for AI model training or complex data center tasks, the A40 will be the better choice.
Leave a Reply
You must be logged in to post a comment.