In the world of deep learning, selecting the right GPU is crucial for achieving the best performance and efficiency. Two prominent contenders in this arena are the NVIDIA RTX A5000 and the Tesla V100-PCIE-16GB. Both GPUs are powerful, but they cater to different needs and budgets. This blog will explore their differences and help you decide which GPU best fits your deep learning requirements.
Introduction to the RTX A5000
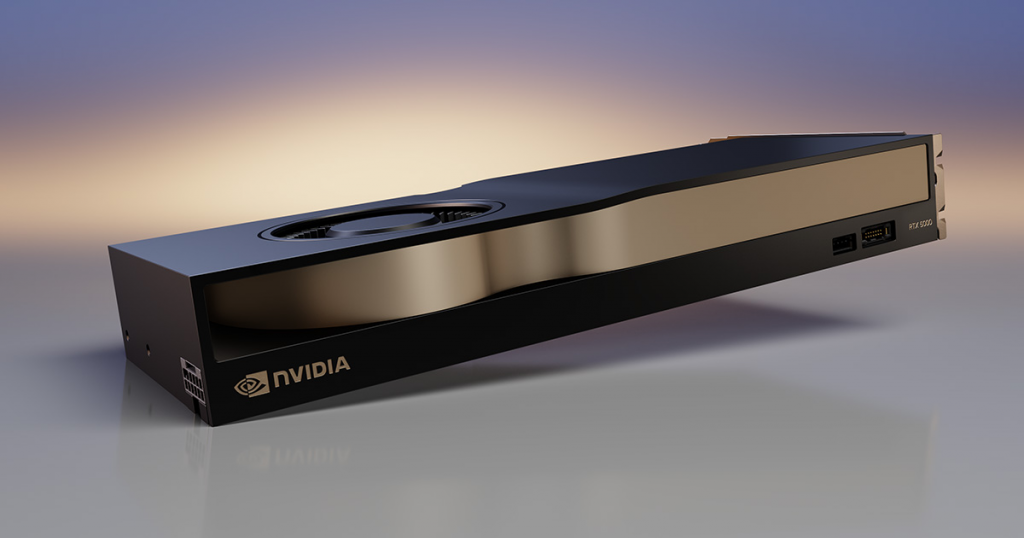
The NVIDIA RTX A5000 represents NVIDIA’s professional GPU line designed to offer a blend of high performance and versatility. Here’s a closer look at its features:
- Architecture: Ampere
- CUDA Cores: 8,192
- Tensor Cores: 256
- Memory: 24 GB GDDR6
- Base Clock: 1.17 GHz
- Boost Clock: 1.73 GHz
- Memory Bandwidth: 768 GB/s
The RTX A5000 is optimized for a variety of AI and deep learning tasks, including both training and inference. Its large memory and robust Tensor Core performance make it suitable for complex models and applications.
Overview of the Tesla V100-PCIE-16GB
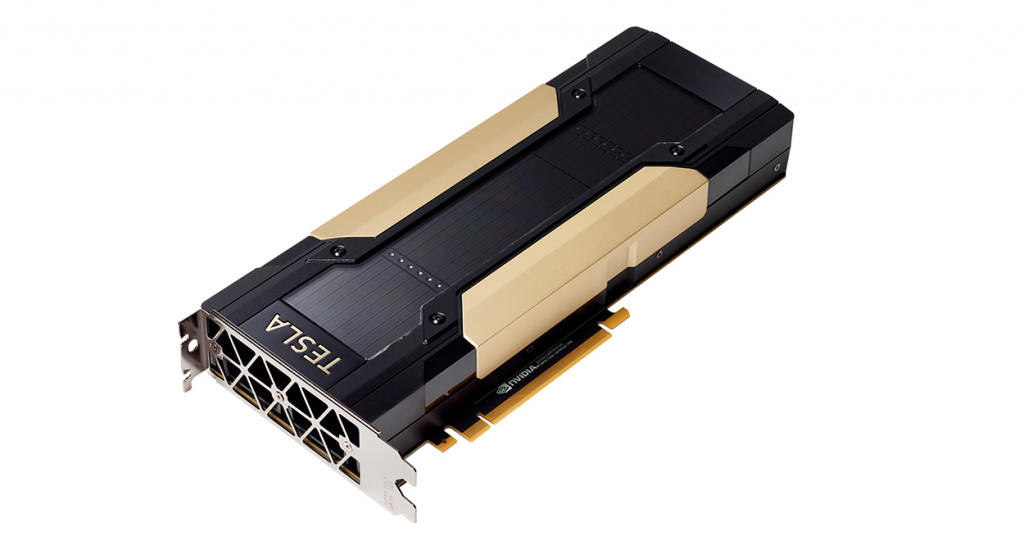
The Tesla V100-PCIE-16GB is a high-performance GPU designed specifically for data centers and demanding computing tasks. Here’s a look at its specifications:
- Architecture: Volta
- CUDA Cores: 5,120
- Tensor Cores: 640
- Memory: 16 GB HBM2
- Base Clock: 1.25 GHz
- Boost Clock: 1.38 GHz
- Memory Bandwidth: 900 GB/s
The Tesla V100 is known for its superior performance in large-scale deep learning tasks, particularly in high-throughput scenarios.
Comparing RTX A5000 and Tesla V100
To facilitate a clearer comparison, here’s a breakdown of their key specifications:
Specification | RTX A5000 | Tesla V100-PCIE-16GB |
---|---|---|
Architecture | Ampere | Volta |
CUDA Cores | 8,192 | 5,120 |
Tensor Cores | 256 | 640 |
Memory | 24 GB GDDR6 | 16 GB HBM2 |
Base Clock | 1.17 GHz | 1.25 GHz |
Boost Clock | 1.73 GHz | 1.38 GHz |
Memory Bandwidth | 768 GB/s | 900 GB/s |
Memory Interface Width | 384-bit | 4096-bit |
Peak FP32 Performance | 27.8 TFLOPs | 15.7 TFLOPs |
Peak FP16 Performance | 55.6 TFLOPs | 125 TFLOPs |
Tensor Performance | 222.2 TFLOPs | 125 TFLOPs |
Total Graphics Power | 230W | 250W |
Power Supply Recommendation | 750W | 800W |
Cooling | Active (Fan) | Passive (Heatsink) |
Interface | PCIe 4.0 | PCIe 3.0 |
NVLink Support | No | Yes |
Precision Supported | FP32, FP16, INT8, TF32, BFLOAT16 | FP64, FP32, FP16, INT8, TF32, BFLOAT16 |
DirectX | 12 Ultimate | N/A |
CUDA Compute Capability | 8.6 | 7.0 |
Form Factor | Dual-slot | Dual-slot |
Target Market | Workstations, AI Development, Rendering | Data Centers, High-Performance Computing |
Price Range (at launch) | $2,500 USD | $8,000 – $10,000 USD |
Architectural Highlights
The RTX A5000’s Ampere architecture brings several enhancements over its predecessor. The upgraded Tensor Cores improve performance for mixed-precision calculations, and the latest AI advancements make it a versatile option for modern workloads. In contrast, the Tesla V100’s Volta architecture was groundbreaking at its release, introducing Tensor Cores that significantly boosted deep learning performance. Despite its age, the V100’s HBM2 memory offers higher bandwidth, beneficial for high-throughput applications.
Performance for Deep Learning
The Tesla V100 is renowned for its top-tier performance in large-scale training tasks, owing to its high number of Tensor Cores and memory bandwidth. It excels in handling complex models and large datasets. The RTX A5000, while not as powerful as the V100, offers excellent performance for a broader range of tasks and provides a better balance of power and cost.
Memory and Bandwidth Considerations
Memory is crucial in deep learning, with the RTX A5000 providing 24 GB of GDDR6 memory, which is advantageous for larger models. However, the Tesla V100’s 16 GB of HBM2 memory offers higher bandwidth (900 GB/s vs. 768 GB/s), which is important for tasks requiring rapid data access.
Software and Ecosystem Support
Both GPUs benefit from NVIDIA’s extensive software support, including CUDA, cuDNN, and TensorRT. The RTX A5000, with its newer architecture, enjoys the latest updates and optimizations. The Tesla V100, though older, remains a reliable choice with well-established support for major deep learning frameworks.
Scalability and Multi-GPU Configurations
For large-scale training, the Tesla V100’s support for NVLink enables high-speed communication between multiple GPUs, ideal for data parallelism. The RTX A5000 supports multi-GPU setups via PCIe, which, while slower than NVLink, is still effective for many applications. The A5000’s cost-effectiveness can be a significant advantage in scaling deep learning workloads.
Power Efficiency and Cooling
The RTX A5000 offers a lower power consumption (230W TDP) compared to the Tesla V100 (250W TDP), potentially reducing operating costs. Its cooling system is generally easier to manage in typical workstation environments, while the V100’s advanced cooling is suitable for data centers and multi-GPU configurations.
Longevity and Future-Proofing
The RTX A5000, being a newer model, is likely to receive longer support for future software updates and optimizations. The Tesla V100, while older, continues to be relevant for its specialized strengths and remains a solid choice for demanding environments.
Price-to-Performance Ratio
The RTX A5000 offers a strong price-to-performance ratio, making it an attractive option for those who need powerful performance without the higher cost of the Tesla V100. The V100’s higher cost is justified by its exceptional performance for large-scale and high-precision tasks.
Suitable Applications
- Researchers and Academics: The RTX A5000’s performance and cost-effectiveness make it ideal for research and academic applications.
- Startups and Small Businesses: For companies starting in AI, the A5000 provides powerful capabilities at a more affordable price.
- Large Enterprises: The Tesla V100 is better suited for enterprises with extensive deep learning needs, offering unmatched performance for large-scale tasks.
Conclusion
Both the NVIDIA RTX A5000 and Tesla V100-PCIE-16GB are formidable GPUs with distinct advantages. The RTX A5000 provides a compelling mix of performance, memory, and cost, making it suitable for a wide range of users. The Tesla V100, with its superior performance for large-scale tasks, remains a top choice for high-demand environments. Your choice will depend on your specific needs, including budget, scale, and the types of models you plan to train.
By carefully considering these factors, you can select the GPU that best aligns with your deep learning goals, ensuring optimal performance and value for your investment.
Leave a Reply
You must be logged in to post a comment.