The Critical Choice for AI Pioneers
Imagine this: you’re on the verge of developing a groundbreaking AI model, and the success of your project hinges on the right hardware. The NVIDIA A5000 and A4000 GPUs, both part of the Ampere architecture, present two compelling options. But which one will propel your AI workload to new heights?
In this blog, we’ll delve into the features, strengths, and differences between these two GPUs to help you make an informed decision tailored to your AI needs.
Why GPUs Are Crucial for Machine Learning and AI
When it comes to accelerating machine learning (ML) and AI tasks, GPUs have become indispensable. They are built to handle the heavy computational demands of these fields, especially when it comes to training complex models on large datasets. The parallel processing capabilities of GPUs allow for quicker data processing, leading to faster and more accurate model training.
NVIDIA has been a leader in this space, consistently pushing the boundaries of what’s possible with GPU technology. The A5000 and A4000 are both designed to meet the high demands of modern AI workloads, but they do so in different ways, making it essential to understand which one best suits your specific needs.
Exploring TensorFlow: A Powerful Tool for AI
TensorFlow is a leading open-source framework for machine learning, widely adopted for building, training, and deploying AI models. Its ability to run efficiently on GPUs makes it a favorite among data scientists and AI developers. TensorFlow’s compatibility with NVIDIA GPUs like the A5000 and A4000 ensures that users can leverage GPU acceleration to significantly speed up model training and inference.
TensorFlow’s performance on GPUs is further enhanced by NVIDIA’s CUDA and cuDNN libraries. CUDA allows TensorFlow to offload computationally intensive tasks to the GPU, while cuDNN provides optimized routines specifically for deep neural networks. This combination of TensorFlow with NVIDIA’s hardware and software stack enables developers to push the limits of AI research and development.
The Technical Breakdown: NVIDIA A5000 vs. A4000
Both the NVIDIA A5000 and A4000 GPUs are based on NVIDIA’s Ampere architecture, but they offer different levels of performance and capabilities. Here’s a breakdown of their key specifications:
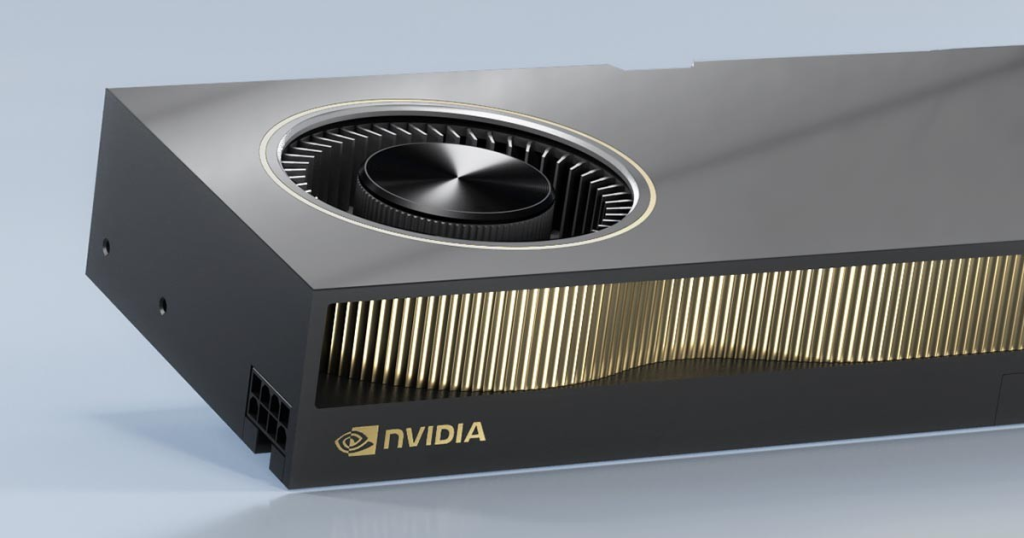
NVIDIA A5000:
- CUDA Cores: 8,192
- Tensor Cores: 256
- Memory Size: 24 GB GDDR6
- Memory Bandwidth: 768 GB/s
- Max Power Consumption: 230W
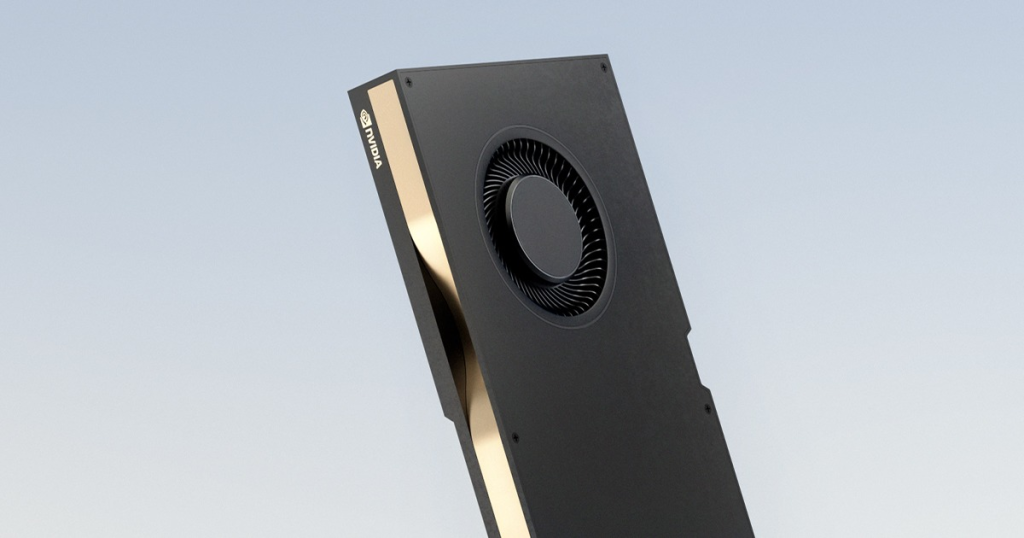
NVIDIA A4000:
- CUDA Cores: 6,144
- Tensor Cores: 192
- Memory Size: 16 GB GDDR6
- Memory Bandwidth: 512 GB/s
- Max Power Consumption: 140W
Both GPUs offer impressive specs, but the A5000 clearly stands out with more CUDA and Tensor cores, greater memory capacity, and higher memory bandwidth, making it the more powerful option for demanding AI tasks.
A Deep Dive into Their Capabilities
Parallel Processing Power
GPUs like the A5000 and A4000 excel in parallel processing, a crucial feature for AI workloads. The A5000, with its higher number of CUDA cores, is better equipped for handling complex computations and larger datasets. This makes it particularly suitable for tasks that require extensive data parallelism, such as training deep learning models.
Memory Capacity and Bandwidth
Memory capacity and bandwidth are critical when dealing with large datasets and intricate models. The A5000’s 24 GB of memory and 768 GB/s bandwidth allow it to manage larger datasets more efficiently, reducing the time needed for data transfers and increasing the overall speed of AI workflows. The A4000, while offering less memory, still provides substantial capacity for smaller to mid-sized datasets.
Power Consumption and Efficiency
Power efficiency is a key consideration, especially for large-scale deployments. The A4000’s lower power consumption makes it an attractive option for environments where energy costs or thermal management are concerns. The A5000, on the other hand, demands more power but justifies it with superior performance.
Benchmarking Performance: Which GPU Wins?
When it comes to TensorFlow and other AI applications, benchmarks are essential to understanding how these GPUs perform in real-world scenarios. Here are some performance metrics to consider:
- Processing Speed: The A5000, with its higher CUDA core count and memory bandwidth, typically offers faster processing times, making it ideal for large-scale training tasks.
- Memory Utilization: The A5000’s larger memory size allows for more efficient handling of extensive models and datasets, reducing the need for data to be transferred back and forth between the GPU and system memory.
- Power Efficiency: While the A4000 is more power-efficient, the A5000’s additional performance may outweigh the higher power consumption, depending on the workload.
Conclusion: A5000 or A4000?
The choice between the NVIDIA A5000 and A4000 ultimately depends on your specific needs. If your work involves large-scale AI projects that require maximum computational power, the A5000 is the clear choice. Its superior CUDA core count, memory capacity, and bandwidth make it ideal for intensive tasks like deep learning model training.
On the other hand, if you’re looking for a more cost-effective solution with lower power consumption for smaller-scale projects, the A4000 offers excellent performance at a lower cost. It’s well-suited for tasks that don’t require the full might of the A5000 but still need reliable GPU acceleration.
Both GPUs bring impressive capabilities to the table, ensuring that whichever you choose, you’re equipped to tackle your AI challenges head-on.
Leave a Reply
You must be logged in to post a comment.