As the AI landscape evolves rapidly, the anticipation for NVIDIA’s upcoming H200 GPU is palpable. With substantial advancements expected over the H100, which has already set new standards for AI performance, it’s crucial to understand how these two GPUs compare. Let’s dive into the architectural advancements and performance metrics to discover what makes the H200 a potentially groundbreaking release.
Architectural Evolution: NVIDIA H100 Overview
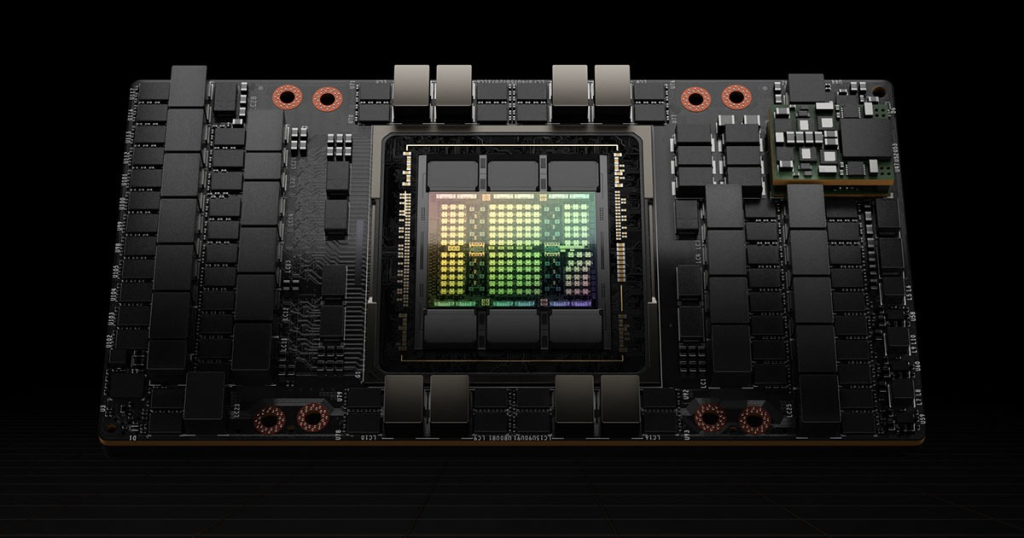
The NVIDIA H100 has been a game-changer in AI and HPC with its robust Hopper architecture. This GPU brought significant improvements over its predecessor, the A100, with higher core frequencies and advanced computational capabilities.
Key features of the H100 include:
- New Streaming Multiprocessor (SM) Design: The H100 features an upgraded SM that enhances execution speed for CUDA threads and floating-point operations.
- FP8 Data Type: Introducing the FP8 format, the H100 achieves faster calculations with slightly reduced precision compared to the FP32 format used in the A100.
- Enhanced SM Architecture: The new SM delivers double the performance for traditional data types and four times the performance boost in floating-point operations over the A100.
The H200: A Leap Forward
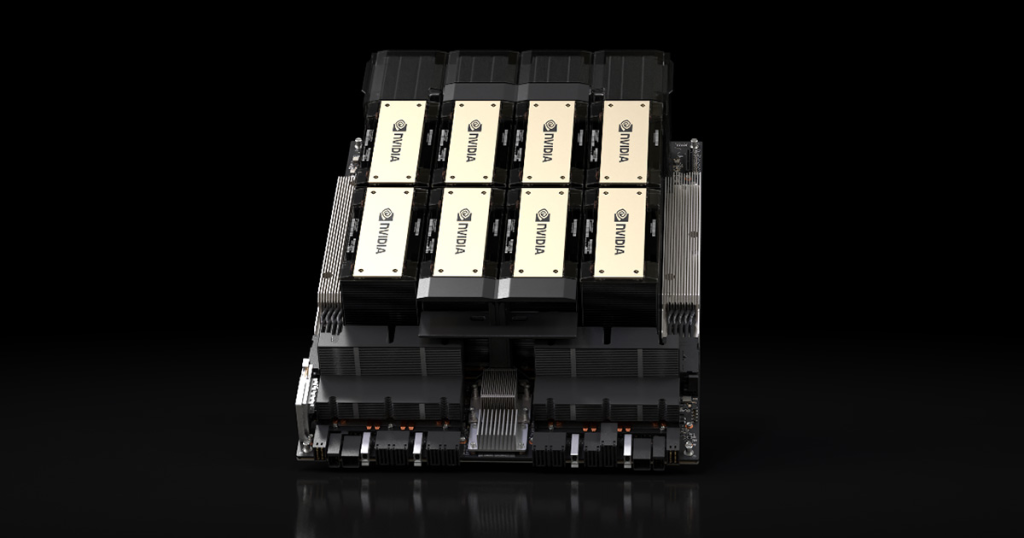
Anticipation for the NVIDIA H200 is high, primarily due to its introduction of HBM3e memory, which brings remarkable advancements:
- Memory Capacity: The H200 offers 141 GB of memory—nearly double that of the H100. This substantial increase allows for larger models and datasets, enhancing the GPU’s capability to manage more complex tasks efficiently.
- Memory Bandwidth: With 4.8 TB/s of bandwidth, the H200 significantly surpasses the H100’s 3.35 TB/s. This improvement accelerates data transfer rates, which is crucial for high-throughput computations.
Comparing Specifications:
Specification | NVIDIA H100 | NVIDIA H200 |
---|---|---|
Form Factor | SXM | SXM |
FP64 Performance | 34 TFLOPS | 34 TFLOPS |
FP64 Tensor Core | 67 TFLOPS | 67 TFLOPS |
FP32 Performance | 67 TFLOPS | 67 TFLOPS |
TF32 Tensor Core | 989 TFLOPS | 989 TFLOPS |
BFLOAT16 Tensor Core | 1,979 TFLOPS | 1,979 TFLOPS |
FP16 Tensor Core | 1,979 TFLOPS | 1,979 TFLOPS |
FP8 Tensor Core | 3,958 TFLOPS | 3,958 TFLOPS |
INT8 Tensor Core | 3,958 TOPS | 3,958 TOPS |
GPU Memory | 80 GB | 141 GB |
Memory Bandwidth | 3.35 TB/s | 4.8 TB/s |
Decoders | 7 NVDEC, 7 JPEG | 7 NVDEC, 7 JPEG |
Max Thermal Design Power | Up to 700W | Up to 1000W |
Multi-Instance GPUs | Up to 7 MIGs @ 10GB | Up to 7 MIGs @ 16.5GB |
Interconnect | NVLink: 900GB/s, PCIe Gen5: 128GB/s | NVLink: 900GB/s, PCIe Gen5: 128GB/s |
Performance Boost: H200 vs H100
The NVIDIA H200’s enhanced memory and bandwidth provide a significant boost in performance, particularly in tasks involving large datasets and complex computations.
Performance Gains:
- Generative AI and HPC Benchmarks: The H200 outperforms the H100 by up to 45% in specific benchmarks due to its superior memory capacity and bandwidth.
- MLPerf Inference Benchmarks: For the Llama 2 70B model, the H200 achieves 31,712 tokens per second in offline scenarios, representing a 42.4% improvement over the H100’s 22,290 tokens per second.
Inference Efficiency:
- Improved Throughput: The H200’s larger memory allows for handling more substantial tasks without frequent data transfers from external memory, resulting in faster and more efficient inference.
- Thermal Management: The H200 maintains comparable power consumption levels while doubling memory bandwidth, effectively reducing the total cost of ownership by 50%.
The Verdict: H100 or H200?
While the H100 has set a high bar with its advanced features and performance, the H200 promises even greater capabilities. Its increased memory, bandwidth, and improved thermal management make it a formidable choice for demanding AI and HPC tasks.
As organizations and researchers continue to push the boundaries of AI, the H200 stands out as a powerful tool for future innovations.
Stay tuned for more updates on the latest in GPU technology, and explore how these advancements can drive your projects forward.
Leave a Reply
You must be logged in to post a comment.