In the rapidly evolving world of artificial intelligence (AI) research, the tools you choose can make or break the efficiency and speed of your work. At the core of these tools are Graphics Processing Units (GPUs), known for their unparalleled ability to process large amounts of data and execute complex computations. Today, we delve into a comprehensive comparison of two formidable GPUs in the market: the GeForce RTX 3090 and the Tesla V100S-PCIE-32GB. Both are powerhouses, yet they cater to different niches within the AI research community.
A Tale of Two GPUs: The RTX 3090 vs. Tesla V100S
Imagine you are working on a project that could redefine AI-driven predictive modeling in healthcare. The stakes are high, the data is vast, and the algorithms are intricate. Your choice of GPU could either accelerate your progress or slow you down. This is where the debate between the RTX 3090 and Tesla V100S comes into play. Both GPUs have earned respect in the field, but which one aligns with your specific needs?
Understanding GPU Architecture: The Building Blocks of AI Performance
GPUs are uniquely designed to handle multiple tasks concurrently, which is critical for the parallel workloads common in AI and machine learning (ML). Key components include CUDA cores (for general-purpose computing), Tensor cores (specialized for AI tasks), and VRAM (Video Random Access Memory). Additionally, memory bandwidth plays a significant role in determining how quickly data can be processed. Both the RTX 3090 and Tesla V100S excel in these areas but with different strengths.
The GeForce RTX 3090: A Closer Look
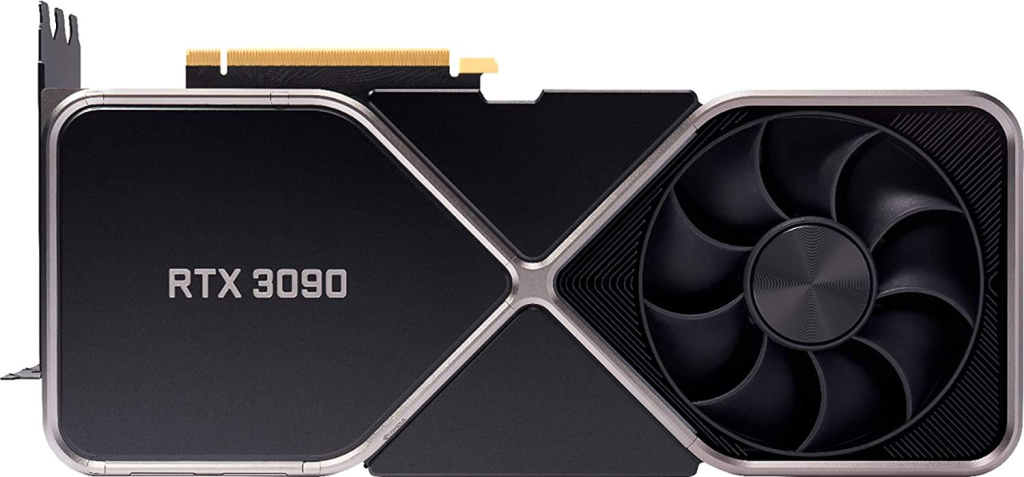
Part of NVIDIA’s Ampere architecture, the GeForce RTX 3090 is equipped with 10,496 CUDA cores, 82 RT cores, and 328 Tensor cores. It features 24GB of GDDR6X VRAM and a memory bandwidth of 936.2 GB/s. While primarily designed for high-end gaming, its hardware is robust enough to handle intensive AI and ML workloads.
Advantages:
- High CUDA and Tensor core counts enable efficient processing of AI tasks.
- Ample VRAM supports large datasets.
- Competitive performance-to-cost ratio for researchers on a budget.
Disadvantages:
- Initially intended for gaming, which might limit certain professional features.
- Higher power consumption and heat generation.
Tesla V100S-PCIE-32GB: The AI Specialist
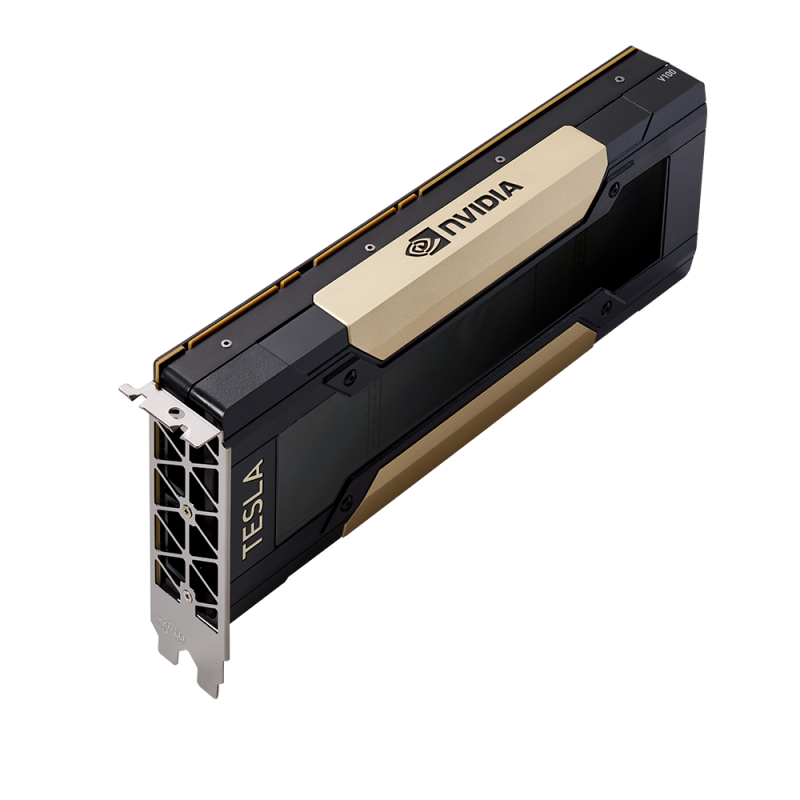
The Tesla V100S, based on NVIDIA’s Volta architecture, comes with 5,120 CUDA cores and 640 Tensor cores. It boasts 32GB of HBM2 VRAM and a memory bandwidth of 1,131 GB/s, making it a specialized tool for scientific computing and AI research.
Advantages:
- Optimized specifically for AI and deep learning tasks.
- Higher memory capacity and bandwidth, ideal for large-scale models.
- More efficient power consumption relative to its performance.
Disadvantages:
- Substantially more expensive than the RTX 3090.
- Not suitable for gaming or non-professional tasks.
Architectural Showdown: Ampere vs. Volta
The Ampere architecture in the RTX 3090 introduces second-generation RT cores and third-generation Tensor cores, improving efficiency and performance. Conversely, the Volta architecture in the V100S is tailored for AI and deep learning, featuring first-generation Tensor cores and superior memory bandwidth.
Tensor cores are particularly significant in AI research as they accelerate matrix operations, which are essential for training neural networks. While both GPUs include Tensor cores, the V100S’s higher count gives it an edge in handling complex AI workloads.
Memory Matters: Bandwidth and Capacity
Memory bandwidth and capacity are critical when dealing with large datasets. The RTX 3090 offers 24GB of GDDR6X VRAM with 936.2 GB/s bandwidth, suitable for many AI tasks. However, the V100S’s 32GB of HBM2 VRAM and 1,131 GB/s bandwidth make it better suited for more demanding applications.
Performance Benchmarks: Numbers That Matter
Benchmark tests often reveal the true capabilities of a GPU. In synthetic benchmarks, the RTX 3090 may score higher in general-purpose tasks, but the V100S shines in AI-specific benchmarks. In real-world AI tasks like neural network training and large-scale simulations, the V100S’s optimized architecture and higher memory bandwidth generally lead to superior performance.
Power Consumption: Efficiency vs. Performance
The RTX 3090 has a thermal design power (TDP) of 350 watts, making it less power-efficient than the V100S, which has a TDP of 250 watts. However, the RTX 3090 offers substantial performance for its power consumption, which may appeal to budget-conscious researchers.
The Software Ecosystem: Support and Tools
Both GPUs support popular AI frameworks like TensorFlow, PyTorch, and Keras. However, the Tesla V100S, being part of NVIDIA’s professional lineup, often receives more tailored optimizations for these frameworks. Additionally, NVIDIA offers a range of developer tools and libraries (e.g., CUDA, cuDNN) that are compatible with both GPUs, though the V100S benefits from additional enterprise-level resources.
Applications: Where Each GPU Excels
GeForce RTX 3090:
- Best for researchers who also engage in gaming or need a versatile GPU.
- Suitable for small to medium-scale AI projects and budget-conscious users.
Tesla V100S-PCIE-32GB:
- Ideal for large-scale AI research, scientific computing, and enterprise-level applications.
- Preferred for projects requiring extensive computational power and efficiency.
Return on Investment: Getting the Most Out of Your GPU
The RTX 3090 offers a high return on investment for projects with limited budgets due to its lower cost and substantial performance. The V100S, though more expensive, justifies its cost for large-scale professional AI research projects with its superior capabilities and efficiency.
Scalability and Flexibility: Adapting to Growing Needs
Both GPUs support multi-GPU configurations, with the RTX 3090 capable of SLI (Scalable Link Interface) setups and the V100S supporting NVLink for higher bandwidth connections. While the RTX 3090 provides flexibility for various use cases, the V100S excels in scalability, particularly for handling large AI models and datasets.
Cooling Solutions: Keeping It Cool
Effective cooling is essential for maintaining GPU performance and longevity. The RTX 3090, with its high power consumption, requires robust cooling solutions, often involving advanced air or liquid cooling systems. The V100S, typically found in data centers, also requires efficient cooling to avoid thermal throttling and ensure consistent performance.
Future-Proofing Your Investment: Looking Ahead
When it comes to future-proofing, the RTX 3090, being a consumer-grade product, may see more frequent updates and releases. On the other hand, the V100S, part of NVIDIA’s enterprise offerings, is designed for long-term use with extended support. Both GPUs are capable of handling emerging AI developments, but the V100S is better positioned for enterprise-level innovations.
User Experience: Ease of Use and Accessibility
The RTX 3090 is relatively user-friendly, with straightforward installation processes suitable for both enthusiasts and researchers. The V100S, designed for data centers, may require more specialized installation but benefits from enterprise-level management tools. Both GPUs come with extensive documentation and community support, making them accessible to researchers and developers alike.
Final Thoughts: Making the Right Choice
Selecting the right GPU for AI research is crucial and depends largely on your specific needs and budget. The GeForce RTX 3090 offers a powerful and cost-effective solution for smaller projects and users who need a versatile GPU. In contrast, the Tesla V100S-PCIE-32GB is the ideal choice for large-scale, professional AI research, delivering unmatched performance, efficiency, and scalability. Understanding the unique strengths and limitations of each GPU will help you make an informed decision that aligns with your research goals.
Comparative Overview: RTX 3090 vs. Tesla V100
Parameter | RTX 3090 | Tesla V100 |
---|---|---|
Architecture | Ampere (2020−2022) | Volta (2017−2020) |
CUDA Cores | 10,496 | 5,120 |
Tensor Cores | 328 | 640 |
Memory | 24GB GDDR6X | 32GB HBM2 |
Memory Bandwidth | 936.2 GB/s | 1,131 GB/s |
TDP (Power Consumption) | 350W | 250W |
Market Segment | Gaming/Desktop | Workstation/Server |
Best Use Cases | Gaming, AI Research | AI, Scientific Computing |
Release Date | September 2020 | March 2018 |
With these insights, you are now better equipped to choose the GPU that will drive your AI research forward, aligning with both your technical requirements and budgetary constraints.
Leave a Reply
You must be logged in to post a comment.