Venturing into deep learning can be directly proportional to feeling overwhelmed by the sheer number of hardware options, especially when it comes to GPUs. It’s like standing at the crossroads of two titans, each promising unparalleled power and efficiency. If you’ve found yourself in this dilemma, you’re not alone. Choosing the right GPU is pivotal, and today, we’ll explore two formidable contenders: the RTX A5000 and the Tesla V100-PCIE-16GB. By the end of this blog, you’ll have a clearer understanding of which one best aligns with your deep-learning aspirations.
The Essential Role of GPUs in Deep Learning
In the rapidly evolving world of deep learning, the GPU is your powerhouse, accelerating the computational processes that make neural networks tick. The speed and efficiency of your deep learning model training depend heavily on the GPU you choose. Understanding GPU specifications is the first step in making an informed decision.
Key GPU Specifications Decoded
Before we dive into the specific GPUs, let’s break down some crucial terms:
- CUDA Cores: These are the cores that perform parallel computations, and more CUDA cores generally mean better performance.
- Tensor Cores: These specialized cores are tailored for deep learning tasks, specifically for tensor operations.
- Memory: VRAM size is essential for handling large datasets without bottlenecks.
- Memory Bandwidth: The rate at which data can be read or written to the GPU.
- TDP (Thermal Design Power): Indicates the GPU’s power consumption and heat output.
These factors collectively determine the efficiency and speed of your deep learning tasks.
Unveiling the RTX A5000: A Modern Marvel
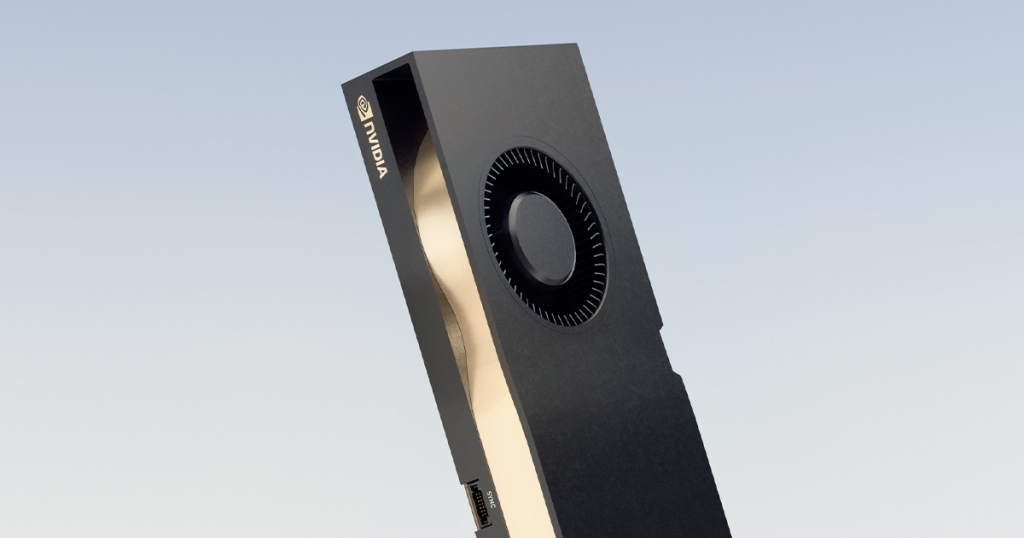
The RTX A5000 is part of NVIDIA’s professional lineup, built on the Ampere architecture. Launched in 2021, this GPU is designed for a range of applications, including deep learning, 3D rendering, and more.
Key Features:
- CUDA Cores: 8192
- Tensor Cores: 256
- Memory: 24 GB GDDR6
- Memory Bandwidth: 768 GB/s
- TDP: 230W
What makes the RTX A5000 stand out is its balance of memory capacity, power efficiency, and versatility, making it a solid choice for many deep learning tasks.
The Powerhouse: Tesla V100-PCIE-16GB
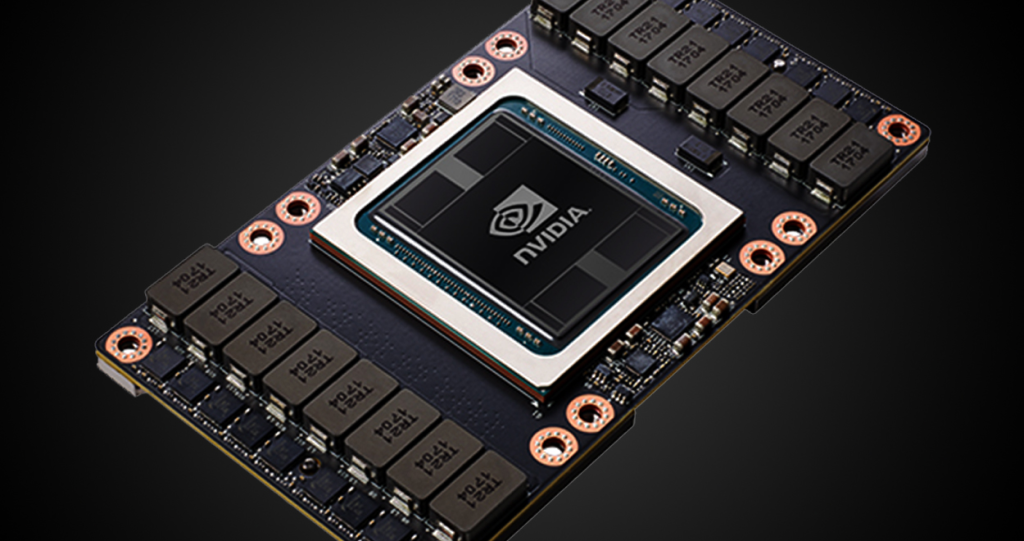
On the other side, we have the Tesla V100-PCIE-16GB, a GPU that has set benchmarks in the AI and deep learning community since its release. Built on NVIDIA’s Volta architecture, this GPU is a go-to for high-performance computing tasks.
Key Features:
- CUDA Cores: 5120
- Tensor Cores: 640
- Memory: 16 GB HBM2
- Memory Bandwidth: 900 GB/s
- TDP: 250W
The Tesla V100 is renowned for its tensor core performance, making it a preferred choice for training large, complex models and running extensive simulations.
Side-by-Side Comparison
Here’s a detailed comparison of the Tesla V100 PCIe and RTX A5000 graphics cards:
Feature | Tesla V100 PCIe | RTX A5000 |
---|---|---|
Architecture | Volta (2017–2020) | Ampere (2020–2022) |
CUDA Cores | 5120 | 8192 |
Tensor Cores | 640 | 256 |
Memory | 16 GB HBM2 | 24 GB GDDR6 |
Memory Bandwidth | 900 GB/s | 768 GB/s |
TDP | 250W | 230W |
Interface | PCIe 3.0 x16 | PCIe 4.0 x16 |
Transistor Count | 21,100 million | 28,300 million |
Process Technology | 12 nm | 8 nm |
DirectX | 12.0 | 12 Ultimate (12_2) |
OpenGL | 4.6 | 4.6 |
Performance Showdown
Tensor Core Strength
The Tesla V100, with its 640 tensor cores, offers unmatched performance in tensor-heavy computations, making it ideal for tasks like training massive neural networks. Meanwhile, the RTX A5000, with 256 tensor cores, provides strong performance for a broader range of tasks, although it lags slightly behind the V100 in tensor operations.
Memory and Bandwidth: The Bigger, The Better?
The Tesla V100 boasts a 900 GB/s memory bandwidth, which allows for faster data transfer. However, the RTX A5000 compensates with a larger 24 GB memory, compared to the V100’s 16 GB. This can be advantageous for models that require extensive datasets.
Power Efficiency
In environments where power consumption is a concern, the RTX A5000 edges out with a TDP of 230W, compared to the Tesla V100’s 250W. This difference might seem minor but can add up in large-scale deployments.
Architecture: Ampere vs. Volta
Ampere: The New Kid on the Block
The RTX A5000 is built on the Ampere architecture, which introduces several new features, including third-generation tensor cores and improved CUDA cores. These enhancements make it highly efficient and capable of handling modern deep-learning tasks with ease.
Volta: The Trailblazer
The Tesla V100, with its Volta architecture, revolutionized deep learning upon its release. Although it’s slightly older, it still holds its ground with specialized tensor cores and high memory bandwidth, particularly for deep-learning models requiring maximum computational power.
Cost and Value
RTX A5000: Bang for Your Buck
For professionals looking for a versatile GPU that won’t break the bank, the RTX A5000 offers excellent value. It balances performance, memory capacity, and power efficiency, making it a solid choice for various deep learning and professional tasks.
Tesla V100: Premium Power
The Tesla V100, though more expensive, delivers superior tensor performance and memory bandwidth, justifying its cost for those who need to push the limits of deep learning and AI research.
Future-Proofing: Which GPU Will Last?
RTX A5000: Built for the Future
With its newer Ampere architecture, the RTX A5000 is better equipped to handle upcoming software updates and evolving deep-learning models, ensuring it remains relevant for longer.
Tesla V100: Still a Contender
Despite being built on the older Volta architecture, the Tesla V100 remains a powerhouse. However, as newer architectures emerge, it may gradually lose its edge over time.
Use Cases
Best Use Cases for RTX A5000
- Data Science Workstations: Ideal for professionals who need a flexible GPU for deep learning, 3D rendering, and more.
- Edge AI Deployments: Suitable for scenarios requiring high performance with lower power consumption.
Best Use Cases for Tesla V100-PCIE-16GB
- Large-Scale Deep Learning: Perfect for training complex models and running extensive simulations.
- AI Research Labs: Beneficial for cutting-edge research requiring maximum computational power.
Conclusion
Choosing between the RTX A5000 and Tesla V100-PCIE-16GB ultimately depends on your specific needs and budget. If your work demands top-tier tensor performance and you’re dealing with extensive deep-learning models, the Tesla V100 is your best bet. On the other hand, if you seek a versatile, cost-effective GPU with ample memory and power efficiency, the RTX A5000 will serve you well.
Invest wisely, as the right GPU can significantly accelerate your deep learning projects, pushing the boundaries of what’s possible in the world of AI.
Leave a Reply
You must be logged in to post a comment.