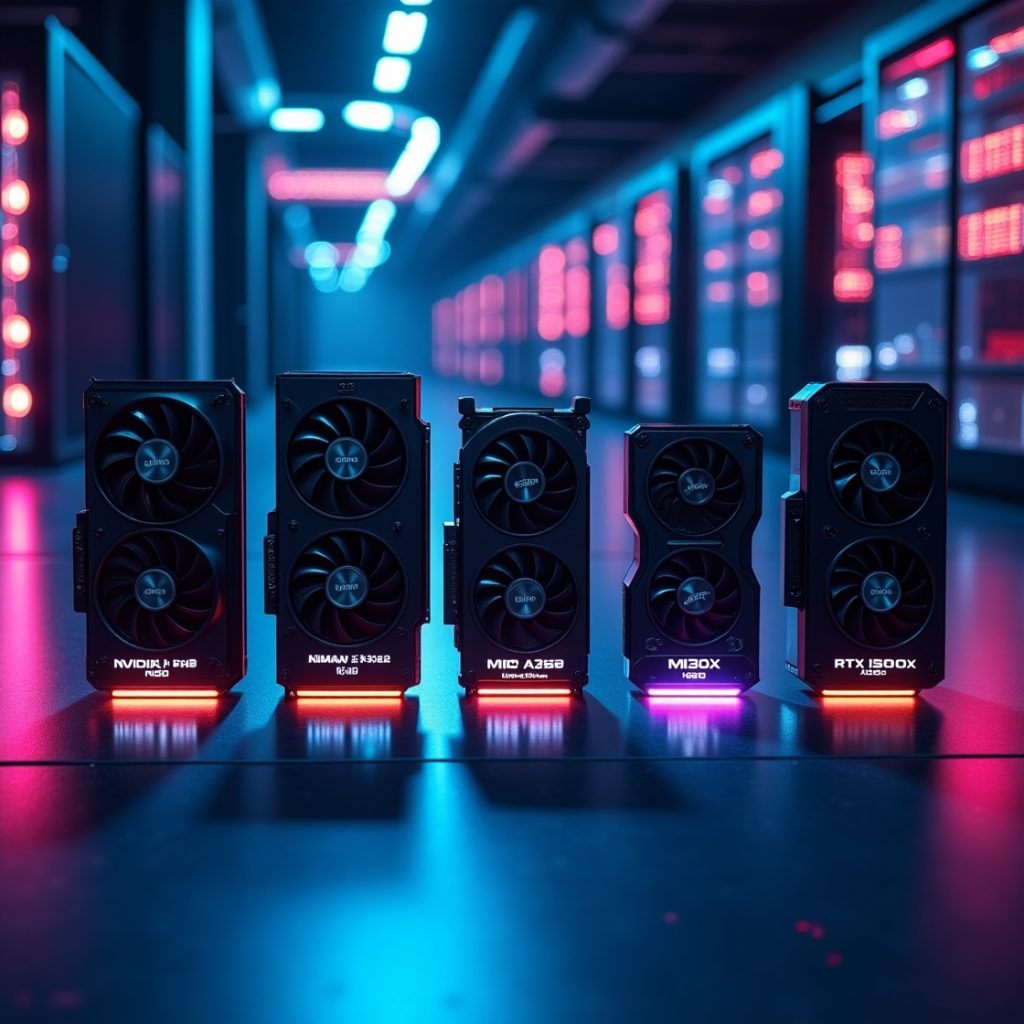
Deep learning workloads demand immense computational power, and choosing the right GPU can significantly impact model training speed, efficiency, and cost. Whether you’re an AI researcher, data scientist, or deep learning enthusiast, selecting the best GPU is crucial. In this guide, we’ll explore the top 5 GPUs for deep learning in 2025, analyzing their specifications, performance, and suitability for AI workloads.
1. NVIDIA H100
Key Features:
- Architecture: Hopper
- CUDA Cores: 16896
- Memory: 80GB HBM3
- Memory Bandwidth: 3.35TB/s
- TDP: 700W
- Tensor Cores: 528
NVIDIA’s H100 is a powerhouse for deep learning, featuring the latest Hopper architecture and significant improvements over the A100. With Transformer Engine optimizations, it excels in training large-scale AI models like GPT-4 and Stable Diffusion. The HBM3 memory and extreme bandwidth make it ideal for enterprise AI applications, cloud-based deep learning, and high-performance computing (HPC).
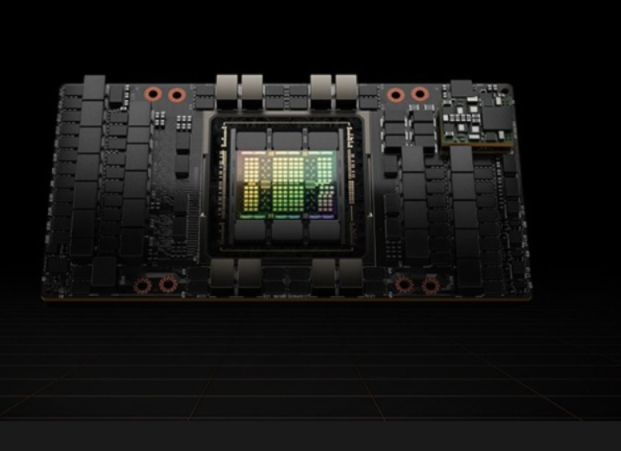
2. NVIDIA A100
Key Features:
- Architecture: Ampere
- CUDA Cores: 6912
- Memory: 80GB HBM2e
- Memory Bandwidth: 2TB/s
- TDP: 400W
- Tensor Cores: 432
The NVIDIA A100 remains a strong contender for deep learning. While the H100 is its successor, the A100 still dominates AI research and inference tasks. It offers multi-instance GPU (MIG) capabilities, allowing efficient workload distribution across multiple users. For those looking for a balance of performance and cost, the A100 is a great alternative to the H100.
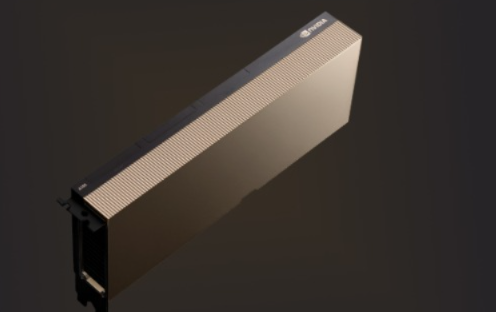
3. NVIDIA H200
While primarily a gaming GPU, the H200 is also widely used for deep learning due to its high CUDA core count and affordability.
Key Features:
- Memory: 48GB HBM3
- CUDA Cores: 18,432
- AI Performance: Strong for entry-level ML tasks
- Price: More budget-friendly than data center GPUs
Why Choose the H200? If you’re an independent researcher or a startup looking for a cost-effective deep learning GPU, the H200 provides strong AI performance at a fraction of the cost of data center GPUs
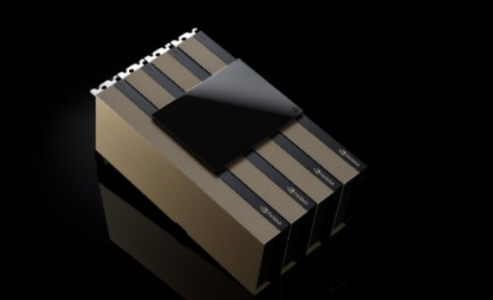
4. AMD Instinct MI300X
Key Features:
- Architecture: CDNA 3
- Compute Units: 896
- Memory: 192GB HBM3
- Memory Bandwidth: 5.3TB/s
- TDP: 750W
AMD’s MI300X is a competitive alternative to NVIDIA’s H100, offering massive HBM3 memory and industry-leading bandwidth. It’s designed specifically for AI training and inference at scale, making it a solid choice for cloud providers and large AI model deployments.
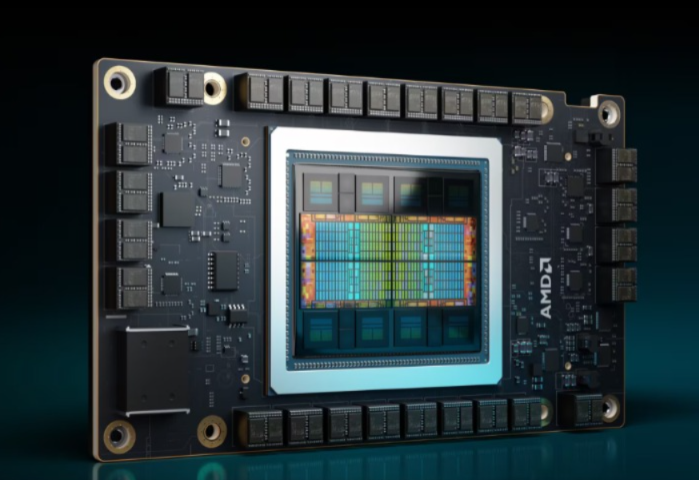
5. NVIDIA RTX 6000 Ada Generation
Key Features:
- Architecture: Ada Lovelace
- CUDA Cores: 18176
- Memory: 48GB GDDR6
- Memory Bandwidth: 960GB/s
- TDP: 300W
The RTX 6000 Ada Generation is a workstation-grade GPU ideal for professional AI research. With ECC memory support and large VRAM, it bridges the gap between consumer and data-center GPUs, making it suitable for training medium-sized AI models without breaking the bank.
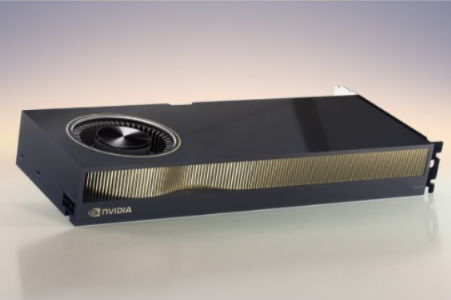
Conclusion
Choosing the right GPU for deep learning depends on your budget, workload size, and computational requirements. Here’s a quick summary:
GPU | Best For |
---|---|
NVIDIA H100 | Enterprise AI & Large Models |
NVIDIA A100 | AI Research & Cloud Workloads |
NVIDIA H200 | AI Training & Inference |
AMD MI300X | High-Bandwidth AI Training |
RTX 6000 Ada | Workstation AI & Research |
Whether you’re training a neural network or fine-tuning a transformer model, selecting the right GPU will greatly enhance your deep learning efficiency and performance in 2025.
Leave a Reply
You must be logged in to post a comment.